The StatTrap III: WAR! What is it good for?
Well… Absolutely Everything
The aim of metric-based player analysis from the beginning was to create an all-encompassing system that valued all of the contributions a player makes to winning games and thus correlated to the team’s success in winning those games. Thus, by proxy, finding the monetary value of that player in relation to run and win contribution. Even before the metrics informed decisions, teams employed slap hitting, automatic out utility infielders. And they often tended to move high powered hitting catchers who can’t frame pitches, are poor at blocking balls, and don’t throw out many base runners to less defensively taxing positions.
Was this the right way to go about doing it? What were the free-agent decisions, roster building and line-up strategies that were being done correctly and what was being done incorrectly? And what was the true translatable value of players across the gamut? The reality of sports is this: we largely know who the true superstars are. The top of the top players are fairly obvious. And arguing the difference in the 4th best guy and the 7th is generally meaningless. We also know who the guys are that are barely hanging on to a roster spot. The true challenge, the make or break, championship building decisions are the 80% in the middle. The weighing of strengths vs deficiencies and the impact of those on the game and the results is the real difference-maker.
Enter WAR -- Wins Above Replacement. One number to summarize total production and contribution that also translates to cost/ value. (For the purposes of simplicity I’m only going to reference positional WAR, you can view a more complete WAR primer here).
While the basic formula for WAR is not that complex:
WAR = (Batting Runs + Base Running Runs +Fielding Runs + Positional Adjustment + League Adjustment +Replacement Runs) / (Runs Per Win)
The machinations to determine run values, however, can be a little more involved both in formula and statistically. And not all systems agree on and use the same inputs. Yet, the premise of each is simply to total the across the board contribution of the player to the wins added over what a “replacement level player” would provide at 0 WAR. This often gets mistakenly referred to as a “hypothetical” player-- but this not actually a hypothetical. So, what then is a replacement level player?
It is essentially any player that could be gotten cheaply from the minor leagues, off waivers or even off-the-street. Basically, a minimum cost player who is readily acquired for virtually nothing at any point and... they absolutely exist. Like in any sport there are “fringe” major leaguers everywhere shuffled onto rosters and into line-ups for various (often injury related) reasons. So, how was replacement level determined? Quite simply, they took all of these players and added up exactly what they produced in their stints in major league baseball, therefore this is a “composite” of the real, actual player performance of “replacements”-- hence Replacement Level Player.
By base-lining the actual production of what these players did with significant data points a true run production value was created for “replacement level”. That baseline could then be fit to teams throughout history who performed at that level (which were basically some of the worst performing teams of all time) to find the win expectation of actual teams who performed at “replacement level”. Thus, providing an actual expectation of both player and win performance for “replacement level”.
From there a regression equation was run to determine that roughly ten runs was the win differential historically between teams. For every ten runs added (scored or prevented) a team won one more game than another team. (For those interested in a more in depth look at this click here ). Hence, ten runs became one win. For every ten runs a player creates or prevents (and this can vary over time to a +/- 1 run range depending on the run scoring environment of the year/ era) they are adding one win to their team. Or +1 WAR vs a “replacement level player” with a baseline 0 WAR.
So what is WAR telling us in practical terms? Say a player has a 5.5 WAR. In an overall general sense what this simply means is that the performance of the player, in all aspects of his play that season, should have produced 55 more runs (or thereabout) than the expectation of a replacement player who is given that same opportunity playing that same position.
Thus, while WAR may have vagaries by what information is put into the equation-- and there can be some subjectivity to that decision and arguments that go either way-- everything inside of the WAR equation is a firmly, logically and objectively defined parameter. We know exactly what constitutes winning baseball in any season or environment and thus can mathematically equate production to wins. Without concrete, factual, translatable information-- you cannot have a WAR system. And for all these fancy machinations and such, this is the elegant simplicity and perfect logic this all comes down to:
Bases Created/ Allowed → Runs Created/ Allowed → Wins/ Losses
Without well defined components across the board, we could not translate production to wins. Going a step further, it allowed for market contracts to be reverse engineered. They took the existing contractual data available and applied it to what teams were actually acquiring in WAR at market by what they were already paying. That then gave us-- what is in present market dollars-- a sum of roughly $8M per win that teams pay in free agency. It has now become a full circle process as many teams pay in free agency by projecting future WAR. A player who can produce or prevent an increment of 10 runs to equal 1 win is worth $8M per win he projects to produce over the life of his contract. Real past market value translated to the system and then reapplied to future market value.
Nothing here is being plucked out of thin air. It is a reconfiguration and refinement of the system, not a re-imaging. And mathematically all of the pieces fit to place as supported by real world knowns and values.
This then fits in precisely with “Pythagorean Records”, another concept devised by Bill James, that correlates teams records to run differential and derives it’s name from it’s resemblance to the Pythagorean Theorem equation:
Pyth records are essentially based on Run Differential and are often used to identify teams who have over or under performed their record and could therefore “fall off the pace” or “make a run” as we know there is strong correlation of Pyth and actual records and therefore it can serve to “smooth out” statistical luck when it comes to wins and losses. The idea here is fairly simple. The more you outscore your opponents, the better you are and the more you are likely to win. By putting away randomness and superstition and the blind luck components, we get down to the raw factors involved. And it’s a concept that pretty well holds universally. Baseball, Football, Basketball, Hockey each now have a “Pyth” or RD record component. And the teams with best season records largely and consistently have the best differentials.
While adding a teams cumulative WAR over the replacement level baseline usually has a strong correlation to actual wins, it has a stronger correlation to pyth records. Why is that?
Because what values in the WAR formula are identifying are the component elements of run scoring. Returning to the basic ideas presented in Moneyball, using OBP over AVG to identify undervalued assets, the elements inside the WAR formula are the production points that historically create or prevent runs-- such as OBP having more weight than standard AVG. As the basic objective of the game is to score and prevent runs we want to weigh how that is done on a consistent basis.
However, in this, we are generally not looking at or counting the actual runs that have been scored/ prevented, we are looking at foundational pieces that create run scoring/ prevention. Why is that of more importance than how many runs teams are actually scoring? Because the process of run scoring can be highly chaotic and is very dependent on sequencing. We don’t want to give weight to incidental occurrences or statistical luck. We are looking for repeatable processes which will eventually pay off over a long enough timeline as has been historically proven. Again referencing OBP, it is a much more stable indicator than batting average which is much more influenced by luck and susceptible to swings.
Here’s a simple example to demonstrate that: a team has three batters in an inning who respectively hit a single, double and triple (assuming other outs in any order before, during and after but that no outs are made on base). Now, if the hits are produced in that order it is assuredly going to score two runs. The triple drives in the other two batters regardless. However, if those hits are produced in a different or reversed order, where the triple precedes the other two hits, there is no assurance the single scores the double or vice versa. It could be an infield hit or sharply one hop lined to a cannon arm outfielder or a slow footed runner trying to score with a bad break....
Now let’s add a home run to the mix. If the home run leads off the inning, there may only be two runs scored. If it comes at the conclusion of the sequence, four runs are scored. The simple order in which hits are accumulated in just a basic scenario showing how sequencing can be the difference between a small and large number of runs in an inning. Even with the exact same hits occurring in those innings.
So how do you control sequencing? You can’t! Simply impossible. There are going to be times that an inning produces one run and times it produces four. The important part to focus on is the players ability to create the bases. The single (or walk), the double, the triple, the home run. The more often those hits happen the more likely you create runs and the more chances you have of maximized sequencing especially with more bases created. You’d rather hit four doubles in an inning than four singles. Which makes you less vulnerable to setbacks or oddities in the sequencing. You’d much rather hit four home runs which all but eliminates any sequencing issue. Thus, the more bases you create-- the more runs you eventually will score. So, that’s what the focus is-- creating bases.
This is, in fact, the process of all team sports: it’s a series of actions that end in a result. In football a 75 yard touchdown drive could commonly include 12 total plays of 0, 0, 0, 2, 3, 4, 6, 7, 8, 12, 15, 18 yards. However, if those plays occur in several of the wrong sequences, that drive stalls out or never even gets started. So, what is your key here? It’s the repeatability of the actions that lead to the right result. The more a team is capable of making those plays, the more opportunities afforded to them, the more often the sequence will fall correctly. The process begets the result. Therefore, the focus is the process. If the process is productive, the result will follow to a reasonable (and predictable) rate.
The two most prevalent analytics right now in football are EP/ EPA and DVOA. These are essentially situational stats based metrics. They are built on the premise that “not all yards are the same”. Four yards gained on 3rd and 3 are much more important than four yards gained on 3rd and 8. One play is more likely to lead to points by gaining a first down, where the other is less likely and could even lead to the opponent scoring points in the next sequence. For an EPA primer click here (http://www.advancedfootballanalytics.com/2010/01/expected-points-ep-and-expected-points.html)
Well, it certainly is true. And both models are data driven metrics collated from historical situations and both derived logically and mathematically. So, therefore it at least begins on the right foot. However, these are “game flow” analytics. And there are several issues here that call into question it’s overall importance and widespread applications along with any attempt at broader use.
First of all, perhaps this stuff is a little too logical. At the end of the day, EPA/ DVOA is kind of basically telling us that making successful plays translates to points. It is a “what” not a “how” or “why” system. The focus is simply the result, that leaves inexplicable ambiguity in it’s noisy and volatile behavior. And the end result tends to simply affirm that the better teams “make more plays”. Sure, they may identify “outlying” teams who are performing under or over their situational play but, unlike run differential, there is nothing that really establishes situational play is actually a skill and therefore a repeatable process and therefore likely to correct in any way. There’s nothing inherently established here that says a team is able to gain more yards than other teams in any particular situation, above and beyond their ability to gain more yards than other teams in any situation-- which is the exact idea these systems are trying to dismiss by the very notion that the simple accumulation of yardage is irrelevant and the importance lies in these situational constructs.
To me these ideas lean heavily toward feeding the “clutch myth”. The idea that certain teams or players can “elevate” their play at certain points of time. For instance, most people would agree that Tom Brady is one of the, if not the, most clutch Quarterback and football player of all-time. So, it naturally follows that Tom Brady elevates his game in the key winning moments. Except he doesn’t.
In fact, in games that come down to the wire, when the game is on the line, Tom Brady gets worse. He doesn’t get better. Historically Brady has a 64.1 comp% and a 1.9% career interception rate. Throughout this time, in “late & close” games his comp% drops to 61.6 and his Int% balloons to 2.5%. In “close games” alone Brady is 62.6% and 2.2%. Not significantly different but notable.
So, where does Tom Brady excel? In blowouts. The substantial number of which he’s been on the winning end of. Brady crushes lesser opponents. And this applies to about every top QB. Over a long enough timeline, players in “clutch” situations usually perform like they do in any other situation. Or relatively closer to it considering the game gets “tighter”. “Clutch” is usually “sample” and perception.
Why is Brady good “in clutch situations”? Well, because he’s pretty damn good most of the time. So even if he’s not quite as good, it’s still very good. It’s just that simple. Therefore, are we really suppose to believe that Brady (or anyone) has a secret ability to create yardage or make plays situationally other than simply his ability to create more yardage and make plays in ALL situations? We already statistically know that isn’t the case. So, why is there a metric evaluating him that way?
DVOA and EPA (and the EPA influenced stats such as QBR) invert the process that sabermetrics used in it’s approach. It’s a top-down, not bottom-up system. Instead of looking at as much information in an overall broader sense-- the whole as sum of it’s parts-- we’re fitting the pieces to create the puzzle. Now that’s fine as far as deconstructing scoring is concerned. But scoring is a result, not an action.
Otherwise, it is simply creating the illusion of a control factor in the sequencing. And while there may be a little more of an opportunity in football to exert some influence, there is nothing established in this that confirms there’s a greater situational skill involved here above and beyond the norm. A team facing 3rd and 12 may throw a screen or a quick slant and the receiver could be tackled after an 8 yard gain as fans groan and commentators ponder the why they didn’t “throw for the sticks”. Well, there is an inherent danger in making that pass. Longer passes downfield are less likely to be completed and more susceptible to leading to turnovers. And, if that receiver slips a tackle and gets those extra 4 yards that result in a first down-- no one groans, no one questions. EPA and DVOA still make that reward… except really for those first 8 yards that are imperative to get those next 4 yards… those were entirely worthless in that purview. The only interest is the positive or negative of the end result.
The very basis of EP/EPA and DVOA are seemingly founded on the principles that sabermetrics set out to debunk and dismiss. In truth, all yards are the same because gaining yardage is a repeatable skill and the very basic essence of the game. Each yard builds toward the next and that is, in fact, the objective at hand. Stringing together yardage, the way baseball players string together bases.
All of this makes it hard to imagine what more could really be done with EPA/ DVOA considering that they are seemingly fairly limited, one track stats. And neither EPA seem to offer any real possibility to build out a WAR like system. The evaluation tools are not nearly comprehensive enough and it’s components aren’t even truly discernible to identify skill. In reality, while only recently popularized, both systems have been around for decades now and no one has really expanded them out much more than what they were when introduced and the player data that is being done is specious at best.
Now some may say the systems fit better to “team” than to “player”. Which is, of course, patently bizarre logic. A team is comprised of it’s players. Any system inherently needs to fit, correspond and translate both ways. If it doesn’t-- how does it have any reliance or value?
And that system must be based on concrete data and correlate to actual results. There’s nothing mystical nor magical going on here. There’s no esoteric secret that only the enlightened can see. The output derives from the positive, neutral and negative input of the players playing the game.
Hearkening back to our previously referenced ESPN Analytics survey, all of this put together, would explain why only 6 of 22 (27%) of the Analytics Insiders selected EPA based analytics as the “most useful metric in the public sphere” while only half that number (3 respondents) selected PFFs Grades/ WAR-- one more than selected PFR’s “Approximate Value” which is about as thumbnail of a sketch “metric” as you can have--- and, in fact, really even has questionable status as a “metric” as it factors in things such as games started and being named Pro Bowl or All-Pro... when they say “approximate” they mean “approximate”. Meanwhile, the largest number of respondents abstained from actually selecting any of those choices as “useful”.
So… now that we have our road map of the history and path taken in baseball analytics and a better sense of where some things have gotten off track and the wrong turns taken in football, we can begin to look at possible ways to get heading in the right direction. Next time….
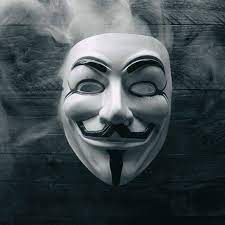